How Geometry, Data and Neighbors Predict Your Favorite Movies — Quanta Magazine
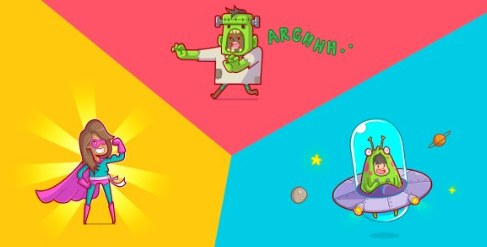
My latest column for Quanta Magazine makes a connection between high school geometry and recommendation engines used by companies like Netflix.
Adrienne is a Marvel movie fanatic: Her favorite films all involve the Hulk, Thor or Black Panther. Brandon prefers animated features like Inside Out, The Incredibles and anything with Buzz Lightyear. I like both kinds, although I’m probably closer to Adrienne than Brandon. And I might skew a little toward Cora, who loves thrillers like Get Out and The Shining.
Whose movie preferences are closest to yours: Adrienne’s, Brandon’s or Cora’s? And how far are your cinematic tastes from those of the other two? It might seem strange to ask “how far” here. That’s a question about distance, after all. What does distance mean when it comes to which movies you like? How would we measure it?
Using the perpendicular bisector–an elementary and underappreciated idea from high school geometry–we can carve up abstract data spaces into regions that can be fruitfully compared and contrasted. And knowing which region you lie in, and whom you are closest to, can help make predictions about your preferences.
To learn more, read the full article, which is freely available here.
3 Comments
Igor P · June 9, 2019 at 4:01 am
I had no idea you weren’t a fan of the oxford comma. When you write out vectors, wouldn’t you separate each independent variable, entity, or attribute with commas? It’s not grammatically incorrect. But I noticed you usually give 3 (or 2?) items in a list. Such as, “Brandon prefers animated features like Inside Out, The Incredibles and anything with Buzz Lightyear.”
Regardless, your column is well written!
This article got me pretty excited. Could be a great exploration into linear algebra. Users can find their locations in space using sums of vectors. They can find the magnitude of their distance from each neighbor by subtraction. And find similarities using dot products!
But people’s preferences change over time. Such change could be tracked to yield a vector field function, where divergence could indicate trends in popular culture. So much to explore!
David Leppik · August 31, 2019 at 7:50 pm
Nice description of Voronoi diagrams, although it actually doesn’t have much to do with how Netflix recommends movies.
Back in the 1990s, I was part of Project Grouplens at the U of MN, which pioneered collaborative filtering. We used a neighborhood model, similar to the one described in that article (Pierson correlation with each movie’s 1-5 star rating being a dimension.) This worked well enough, despite some glaring problems relating to how people rate things. For example, people almost never give 1-star ratings, and people are inconsistent in how they choose 4 or 5 star ratings. In fact, people avoid movies they think they won’t like, so just seeing a movie implies they are either interested in similar movies or it was such a hit they couldn’t avoid it (e.g. Star Wars.)
A few years later I was at Grouplens’ commercial spin-off, which sold collaborative filtering technology to Amazon and other dot-com startups. We had lots of people (not myself, for the most part) trying to improve the technology. After applying all sorts of math we came to the conclusion that you might as well just ignore neighborhoods and look at how often people who see movie A also see movie B. (Or purchase products A and B together, etc.) That is, you compute the frequency with which A and B appear together. Then for each movie you remember the 10 most related movies. When a person asks for recommendations, you combine the top-10 lists for all the movies they’ve seen. This approach provides about 80% accuracy and can work really fast.
This is why Amazon shows you items that people tend to buy together. It’s easy to explain, fast to calculate, and works pretty well. It’s probably also how Netflix first did personalized ratings.
Then about a decade ago, Netflix had a contest (the “Netflix Prize”) for computing more accurate ratings. Turns out it’s really tricky, and I was really skeptical that they could reach their million-dollar target (90% I think.) There are lots of things that have to be taken into consideration. People’s tastes change over time. When someone watches a real stinker, the next decent movie is 5-star by comparison. And so on. They managed it, but with a hybrid approach where the closest teams joined together and used a weighted average of their best algorithms.
Netflix probably uses a simplified version of the Netflix Prize winner. Although these days they don’t give 5-star recommendations. Turns out that convincing you to watch something is a much different problem than determining whether you’re likely to enjoy it.
MrHonner · September 1, 2019 at 9:33 am
Thanks for the reply, and for all the details. It seems like the answer in production is always something much simpler (yet elegant in its own way) than what the mathematicians design. Maybe another issue is that the original approach creates an complex and expensive space to explore all kinds of different relationships, whereas if it’s just one relationship you care about, something cheaper and more narrowly focused can work.
Thanks again for the comment, and for giving me some new things to think about! I appreciate this short history from an insider’s perspective.